- Sat 10 October 2020
- Publication
- Link
Deep Integration of Physical Humanoid Control and Crowd Navigation
Brandon Haworth, Glen Berseth, Seonghyeon Moon, Petros Faloutsos, Mubbasir Kapadia | |
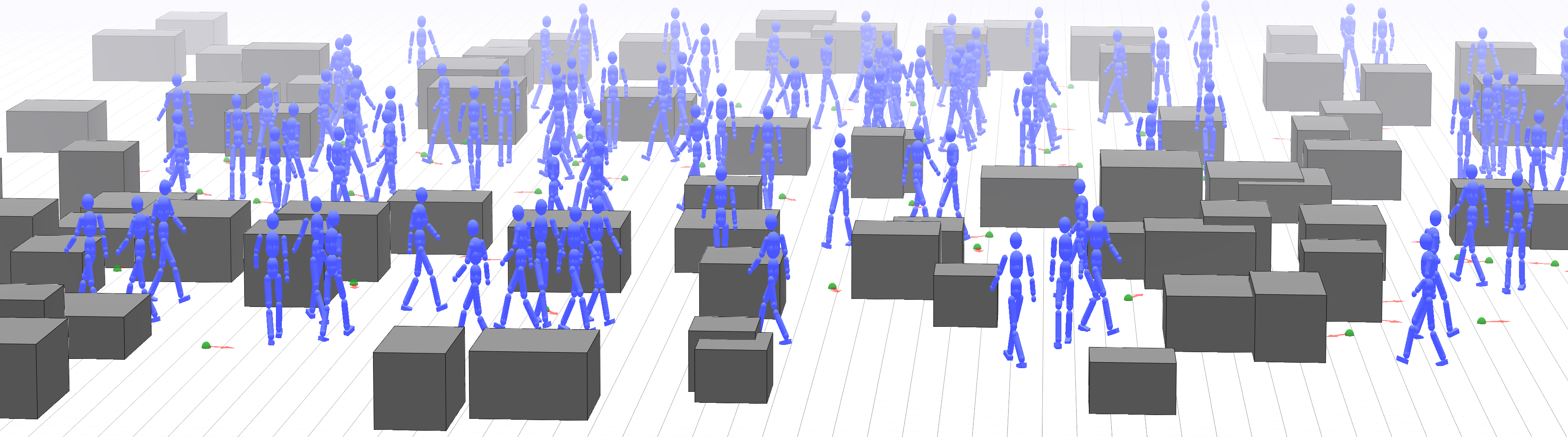
Many multi-agent navigation approaches make use of simplified representations such as a disk. These simplifications allow for fast simulation of thousands of agents but limit the simulation accuracy and fidelity. In this paper, we propose a fully integrated physical character control and multi-agent navigation method. In place of sample complex online planning methods, we extend the use of recent deep reinforcement learning techniques. This extension improves on multi-agent navigation models and simulated humanoids by combining Multi-Agent and Hierarchical Reinforcement Learning. We train a single short term goal-conditioned low-level policy to provide directed walking behaviour. This task-agnostic controller can be shared by higher-level policies that perform longer-term planning. The proposed approach produces reciprocal collision avoidance, robust navigation, and emergent crowd behaviours. Furthermore, it offers several key affordances not previously possible in multi-agent navigation including tunable character morphology and physically accurate interactions with agents and the environment. Our results show that the proposed method outperforms prior methods across environments and tasks, as well as, performing well in terms of zero-shot generalization over different numbers of agents and computation time.