- Wed 02 October 2019
- Publication
Interactive Architectural Design with Diverse Solution Exploration
Glen Berseth, Brandon Haworth, Muhammad Usman, Davide Schaumann, Mahyar Khayatkhoei, Mubbasir Turab Kapadia, Petros Faloutsos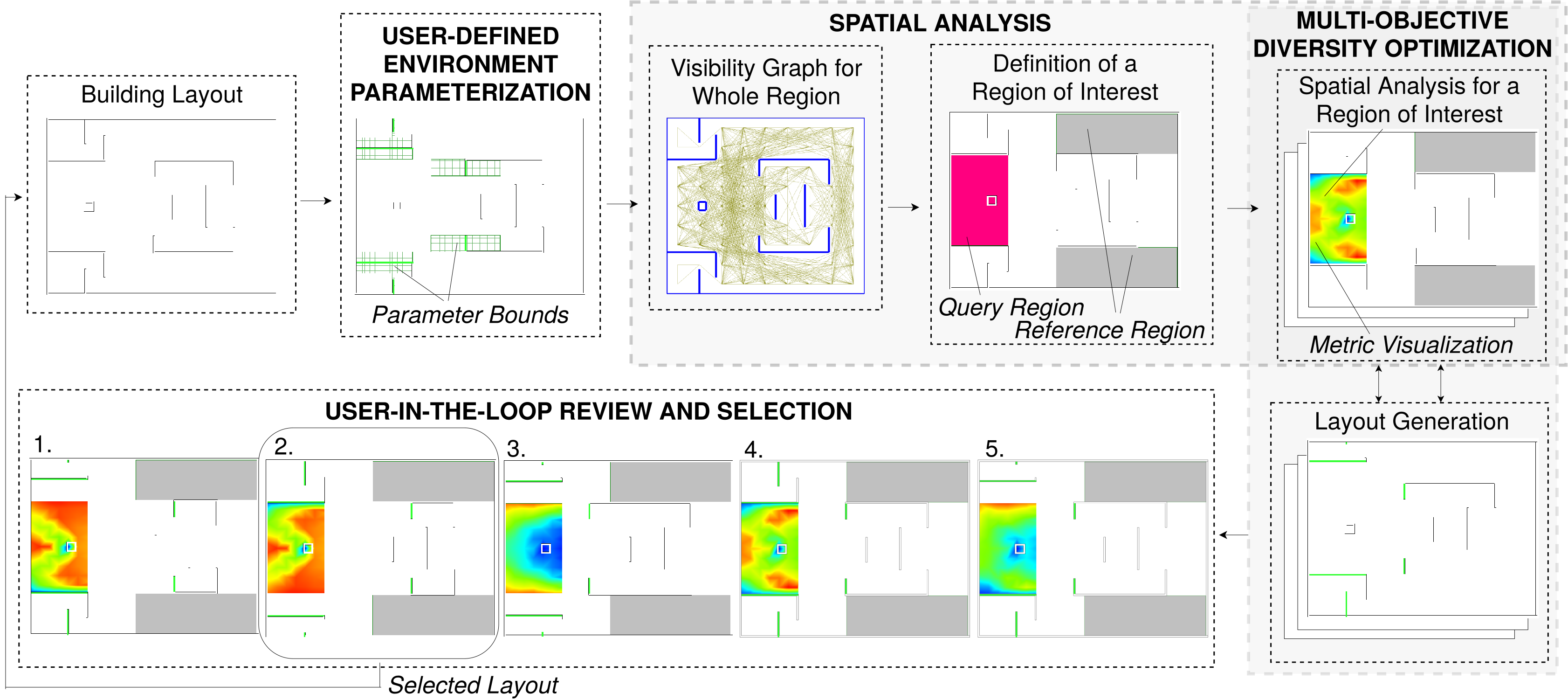
In architectural design, architects explore a vast amount of design options to maximize various performance criteria, while adhering to specific constraints. In an effort to assist architects in such a complex endeavour, we propose IDOME, an interactive system for computer-aided design optimization. Our approach balances automation and control by efficiently exploring, analyzing, and filtering space layouts to inform architects' decision-making better. At each design iteration, IDOME provides a set of alternative building layouts which satisfy user-defined constraints and optimality criteria concerning a user-defined space parametrization. When the user selects a design generated by IDOME, the system performs a similar optimization process with the same (or different) parameters and objectives. A user may iterate this exploration process as many times as needed. In this work, we focus on optimizing built environments using architectural metrics by improving the degree of visibility, accessibility, and information gaining for navigating a proposed space. This approach, however, can be extended to support other kinds of analysis as well. We demonstrate the capabilities of IDOME through a series of examples, performance analysis, user studies, and a usability test. The results indicate that IDOME successfully optimizes the proposed designs concerning the chosen metrics and offers a satisfactory experience for users with minimal training.